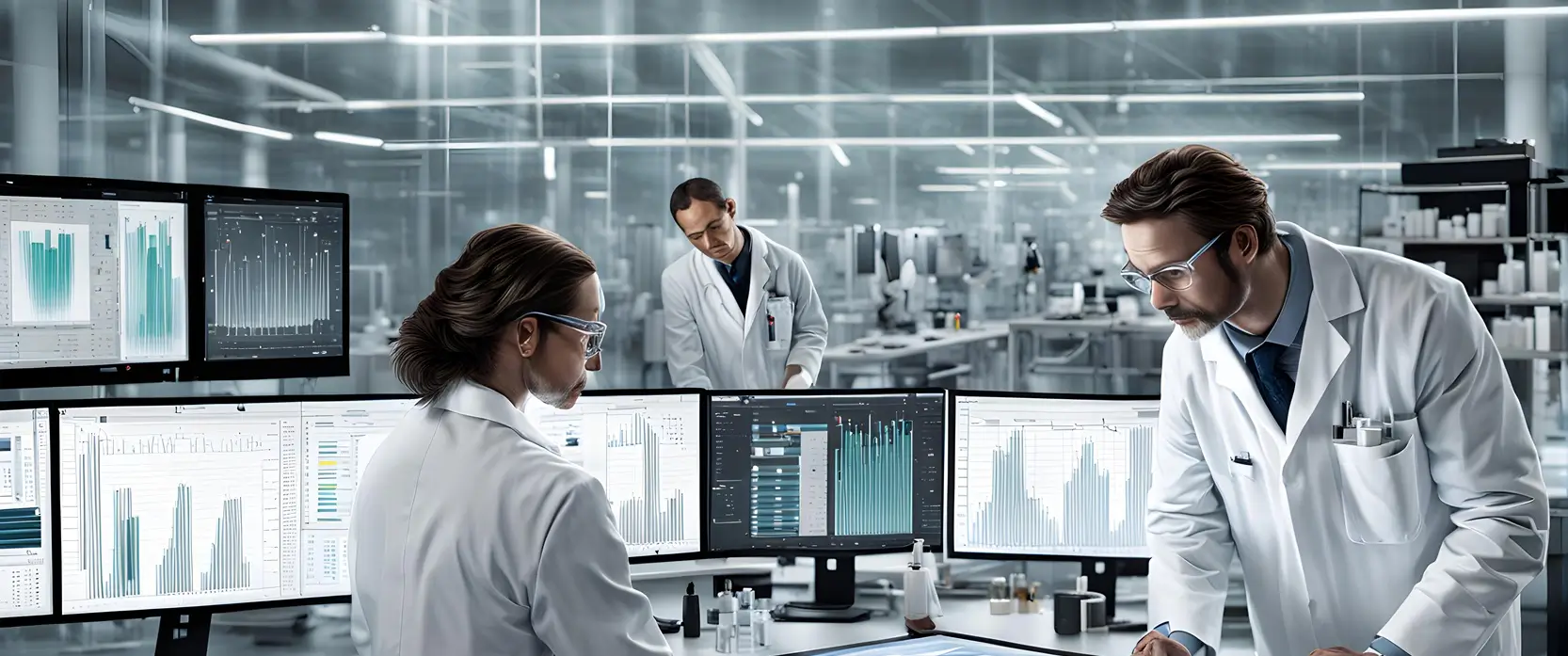
Dispersion, a fundamental process across various industries, plays an important role in shaping the properties of materials. Achieving high-quality dispersion is not merely a goal but a necessity for optimizing outcomes in fields ranging from pharmaceuticals to electronics.
The foundation of assessing dispersion quality lies in the data acquired during the process. Measurements encompass a spectrum of parameters, including particle size, distribution, Zeta potential, and concentration. Accurate data collection forms the backbone of meaningful analysis, ensuring that the subsequent calculations and interpretations are built on reliable information, prioritizing precision in measurement techniques to guarantee the integrity of the data being analyzed.
Calculations for Dispersion Quality
In the quantitative assessment of dispersion quality, a multitude of calculations comes into play. Techniques like dynamic light scattering or laser diffraction serve as invaluable tools to explore particle size distribution, shedding light on the range and uniformity within a medium. Concurrently, concentration calculations, whether in weight or volume percentages, furnish a meticulous quantitative foundation for understanding the dispersion’s characteristics. To grasp a comprehensive understanding, the key lies in the precise quantification of particle size distribution analysis. Dynamic light scattering and laser diffraction, grounded in the principles of light scattering, stand out, enabling the characterization of particles within the nanometer to micrometer range. The Brownian motion of dispersed particles induces fluctuations in scattered light, offering a dynamic profile of particle sizes.
Dynamic light scattering employs a mathematical representation to calculate the intensity autocorrelation function, denoted as q(τ). This function is crucial for analyzing the behavior of particles in a sample. The formula, q(τ)=1N∑i=1Ai⋅cos(ωiτ+ϕi), breaks down as follows: N is the particle count, Ai signifies particle amplitude, ωi is the angular frequency of the ith particle, and ϕi represents the phase shift. This comprehensive formula enables a detailed examination of particle dynamics, providing insights into their characteristics and interactions within the system.
The Laser Diffraction Formula, D(h) = sin(θ) * λ, encapsulates essential parameters for particle analysis. Here, D(h) denotes the particle diameter, λ signifies the wavelength of the laser light, and θ represents the angle of scattering. The formula’s simplicity hides its significance in clarifying particle size based on the diffraction pattern created by laser light interacting with the particles. By understanding these elements,
researchers can derive precise information about particle dimensions, aiding various fields from materials science to environmental monitoring.
Concentration calculations also play an important role in evaluating dispersion quality, offering quantitative insights into the concentration of the dispersed phase within a medium. For the Weight Percentage (% w/w) Calculation, the formula is (% w/w) = (Weight of dispersed phase / Total weight of dispersion) * 100. Similarly, Volume Percentage (% v/v) Calculation follows the formula (% v/v) = (Volume of dispersed phase / Total volume of dispersion) * 100.
These calculations, whether in weight or volume percentages, serve as invaluable tools for researchers, providing a precise means to monitor and regulate the dispersed material’s quantity, ensuring accuracy in various scientific and industrial applications.
In addition to the techniques mentioned earlier, it is important to consider the critical number of test samples when evaluating dispersion quality. The critical number of test samples can be calculated using the dispersion calculator, which takes into account the coefficient of variation and the number of samples analyzed. This critical number helps ensure the reliability and accuracy of the dispersion analysis, providing valuable insights into the quality of the dispersion.
Furthermore, the dispersion quality calculations are essential not only for scientific research but also for industrial applications. They enable researchers and professionals to monitor and regulate the dispersed material’s quantity with precision, ensuring the accuracy and consistency of various processes and products.
Common Data Analysis Techniques
Navigating dispersion quality requires statistical methods for nuanced data understanding. In the realm of statistical parameters for particle size analysis, the mean particle size (μ) is denoted by the below formula, calculated as the sum of all particle sizes divided by the total number of particles.
μ = (∑i=1^N Particle Size i) / N
The standard deviation (σ), quantifies the spread or dispersion around the mean, signifying a more uniform distribution when σ is smaller.
σ = √(∑i=1^N (Particle Size i – μ)^2 / N)
Variance (σ²), the square of the standard deviation, is a pivotal statistical parameter that encapsulates the overall variability in a dataset.
Calculated as σ² = ∑i=1^N (Particle Size i – μ)^2 / N
It provides valuable insights into the extent of dispersion around the mean. This measure contributes significantly to a nuanced assessment of data spread and distribution, enhancing our understanding of the dataset’s statistical characteristics
Principal Component Analysis (PCA) and Statistical Process Control (SPC) play important roles in dispersion quality analysis. PCA transforms variables into uncorrelated components, revealing intricate relationships, while SPC monitors processes, establishing real-time control limits for adjustments. Both methodologies require advanced statistical software, elevating the sophistication of dispersion quality analysis.
Moving beyond mean and standard deviation, effective data interpretation demands adept pattern recognition and anomaly detection. Identifying trends and irregularities becomes instrumental in unveiling the stability and reproducibility of dispersion processes.
In dispersion quality assessment, the integration of advanced data analysis ensures a holistic approach, combining quantitative evaluation with qualitative understanding. One of the significant advantages of data analysis in dispersion processes is its role in troubleshooting. Identifying deviations from the expected data patterns allows engineers and researchers to pinpoint issues such as agglomeration, inadequate mixing, or inconsistent concentration. Armed with this information, targeted interventions can be implemented to rectify problems and enhance dispersion quality.
Interpretation of Dispersion Characteristics
Upon accurate execution of the specified calculations, the resulting data serves as a quantitative basis for interpreting dispersion characteristics. The revelation of a uniform particle size distribution discerned through techniques like dynamic light scattering or laser diffraction stands as an indicator of effective dispersion. This uniformity suggests an optimal scenario where particles are evenly distributed within the medium.
In contrast, the identification of a skewed distribution raises flags regarding potential challenges, such as agglomeration or uneven mixing.
Upon accurate execution of the specified calculations, the resulting data serves as a quantitative basis for interpreting dispersion characteristics. This uniformity suggests an optimal scenario where particles are evenly distributed within the medium. In contrast, the identification of a skewed distribution raises flags regarding potential challenges, such as
agglomeration or uneven mixing. These irregularities not only impact the immediate properties of the dispersion but can also have cascading effects on downstream processes and applications. It underscores the importance of not only identifying but promptly addressing deviations from the desired uniform distribution, ensuring the reliability and quality of the dispersed system in various contexts.
Concentration calculations play a crucial role in this interpretative process, shedding light
on the proportion of the dispersed phase within the medium.
The meticulous application of mathematical rigor to these calculations ensures that the obtained data transcends mere qualitative observations, providing researchers with quantitative insights into the nuanced aspects of dispersion quality. This quantitative approach proves instrumental in guiding researchers to optimize formulations, as it allows for a more precise understanding of the concentration of dispersed material. In essence, the combination
of particle size distribution analysis and concentration, calculations form a stable foundation for comprehensive interpretation, enabling researchers to navigate and enhance dispersion characteristics with a heightened level of precision.
Real-world Applications
In the pharmaceutical industry, achieving precise dispersion of drug particles within a solvent is paramount for optimizing bioavailability and therapeutic efficacy. Data analysis techniques, particularly focused on particle size distribution, enable researchers to fine-tune formulations. By interpreting the data, scientists can ensure that the dispersion aligns with targeted specifications, influencing factors such as dissolution rates and overall drug performance. Real-time monitoring of these parameters
through data analysis facilitates adjustments in formulations, leading to enhanced drug delivery systems.
In materials science and engineering, dispersion is critical in developing advanced materials with tailored properties. The careful distribution of particles within matrices, guided by scientific principles, results in materials exhibiting enhanced mechanical properties and overall performance. Data analysis techniques aid in precisely characterizing these dispersions, allowing researchers to correlate dispersion
quality with material functionality. Such insights build the designs of aerospace, automotive, and electronics materials, driving innovation and efficiency.
Even in the food industry dispersion quality is a sensory and functional cornerstone. The even distribution of flavoring agents, emulsifiers, and stabilizers within food products is a science-based art. Data analysis of concentration and particle size distribution ensures the harmonious blending of ingredients, influencing both the texture and taste of a wide array of products.
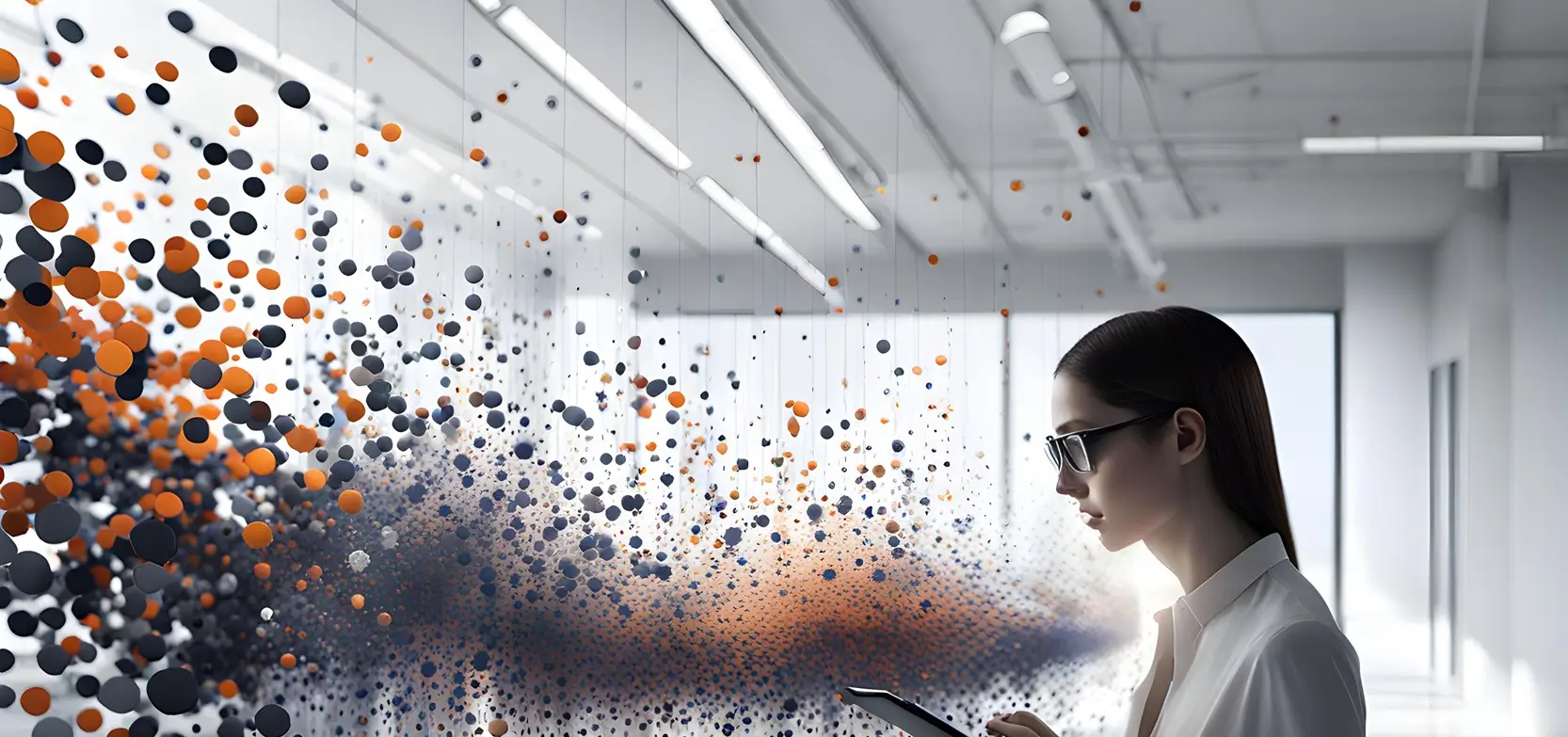
Through meticulous interpretation of this data, food scientists can consistently deliver delightful culinary experiences, from chocolate bars to carbonated beverages.
Full potential
The ability to harness the full potential of data analysis in dispersion processes not only ensures stability and uniformity but also unlocks opportunities for innovation and problem-solving. The continuous quest for perfection in dispersion, guided by data-driven insights, propels industries forward. As we navigate this intricate chemical domain, from agglomeration to irreversible aggregation, data analysis stands as the guiding light that illuminates the path toward optimal dispersion quality.